What is data discovery?
Data discovery is a dynamic process that involves exploring, classifying, and analyzing data from diverse sources to uncover patterns and extract actionable insights. It allows users to visually navigate data relationships and apply advanced analytics to optimize business decisions and performance.
Data discovery identifies what data exists and how it can be strategically used to drive value and efficiency within an organization.
By simplifying the torrent of business data into clear, actionable insights, data discovery effectively acts as the linchpin for decisive data-driven action.
Key takeaways
Data discovery is the process of exploring and analyzing diverse data sources to uncover patterns and drive actionable insights, enhancing business decisions and performance.
Data discovery methods include manual processes, which require extensive knowledge of data organization, and automated processes that utilize AI and machine learning to streamline analysis and ensure data reliability.
Data discovery is a cyclical, iterative process comprising goal setting, aggregation, preparation, visualization, analysis, and repetition, enabling continuous refinement and relevance to business objectives.
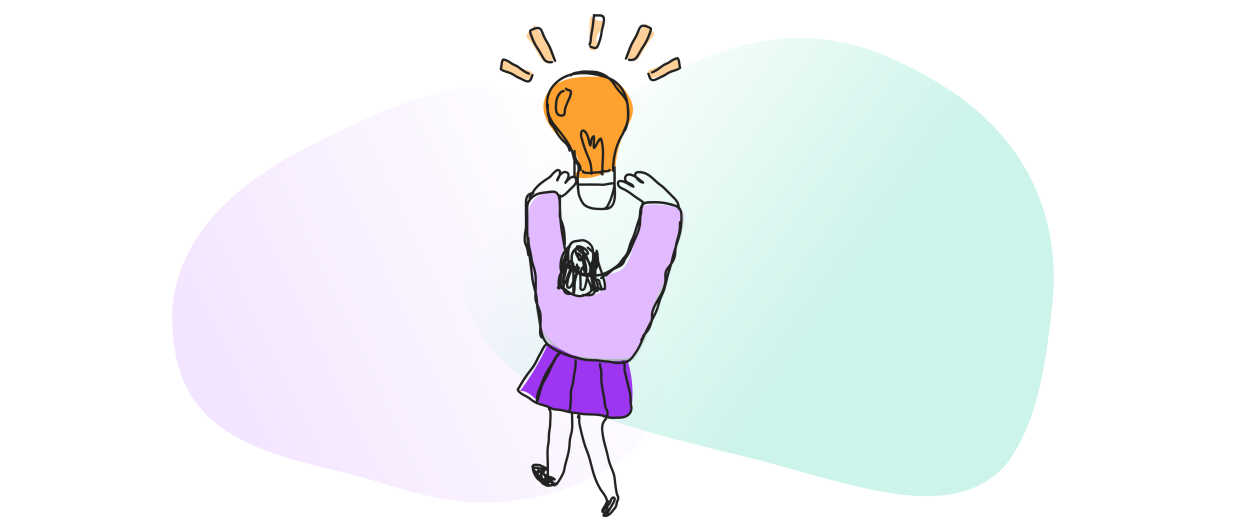
Think of data discovery as a treasure hunt. It’s a process that involves:
Collecting, cataloging, and sifting through data to unearth valuable insights
Driving informed decision-making and improving business performance
Visually navigating through data
Applying advanced analytics to detect patterns and gain insights
Data discovery empowers teams by facilitating what Forbes highlights as the leading trend in data analytics: democratization. This trend allows everyone in an organization to explore and comprehend data easily as well as:
Bridging all areas of your business
Uncovering insights hidden within their data
Fostering a culture of collaboration and teamwork
Why data discovery matters
Data discovery plays an essential role in various facets of a business. These include:
Optimizing business processes
Identifying opportunities for cost savings
Ensuring data security and accuracy
Additionally, data discovery fosters AI readiness by creating structured, reliable data that AI models can analyze more effectively. This foundation enables businesses to harness AI tools confidently, driving more accurate insights and innovative applications.
Explore how businesses can leverage data discovery to achieve success.
Better customer experiences
Companies have begun leveraging Voice of Customer programs to improve customer experiences. Some benefits of analyzing customer data include:
Revealing friction points in user journeys
Providing insights for more personalized experiences
Identifying areas for improvement in products or services
By implementing these programs, companies can create more enjoyable customer experiences.
For instance, browsing and purchase history insights can help tailor product recommendations, making shopping feel more personalized and efficient.
Product innovation
Data insights can be a guiding star for product innovation. By understanding user needs and market trends, businesses can:
Develop new products or updates that align with customer expectations
Ensure innovations are timely and resonate with what customers genuinely want
Keep your business competitive
This data-driven approach ensures your business stays ahead of the curve and delivers products that meet customer needs.
Optimized operations
Data discovery highlights operational inefficiencies, enabling companies to streamline processes for cost reduction and enhanced performance.
For example, AI and machine learning automate the creation of metadata repositories, accelerating the understanding of where and how data is utilized across an organization.
Data-informed strategy
A deep dive into market and consumer behavior data can guide strategic choices that resonate with business goals and meet consumer needs. Decisions backed by data are more likely to yield success, from market entry strategies to targeted marketing campaigns. The result? Efforts that are well-directed and effective.
Methods of data discovery
There are two main methods of data discovery: manual and automated. Each method has its strengths, and the choice between the two often depends on the complexity of your data and the resources at your disposal.
Manual
Manual data discovery involves collecting and consolidating data from various sources into a single location. This method is crucial for transforming different data structures into a consistent format for analysis.
Data profiling, the initial step in manual data discovery, identifies which data sources are worth investigating, saving time and improving accuracy.
Visualizations such as charts, graphs, and heatmaps are key to manual and visual data discovery. These visual aids enable the identification of trends and patterns at a glance. However, manual discovery requires extensive data categorization and lineage knowledge to map and organize data effectively.
Automated
Automated data discovery, on the other hand, leverages AI and machine learning to streamline the analysis process. During the preparation phase, automated tools have the capability to:
Erase outliers
Unify data formats
Detect null values
Standardize data quality
These processes are essential for ensuring the reliability of the data being used. This method enables sales teams and business users to find relevant data insights without needing extensive data expertise.
Automated process discovery tools use machine learning and process mining algorithms to track activities within a system, offering an accurate view of business operations.
These tools can automate key steps such as data collection, process mapping, and providing automated insights, significantly accelerating the discovery process and making data discovery processes more efficient.
6 steps in the data discovery process: how is data discovered?
Now that we’ve explored the methods, let’s dive into the mechanics of data discovery.
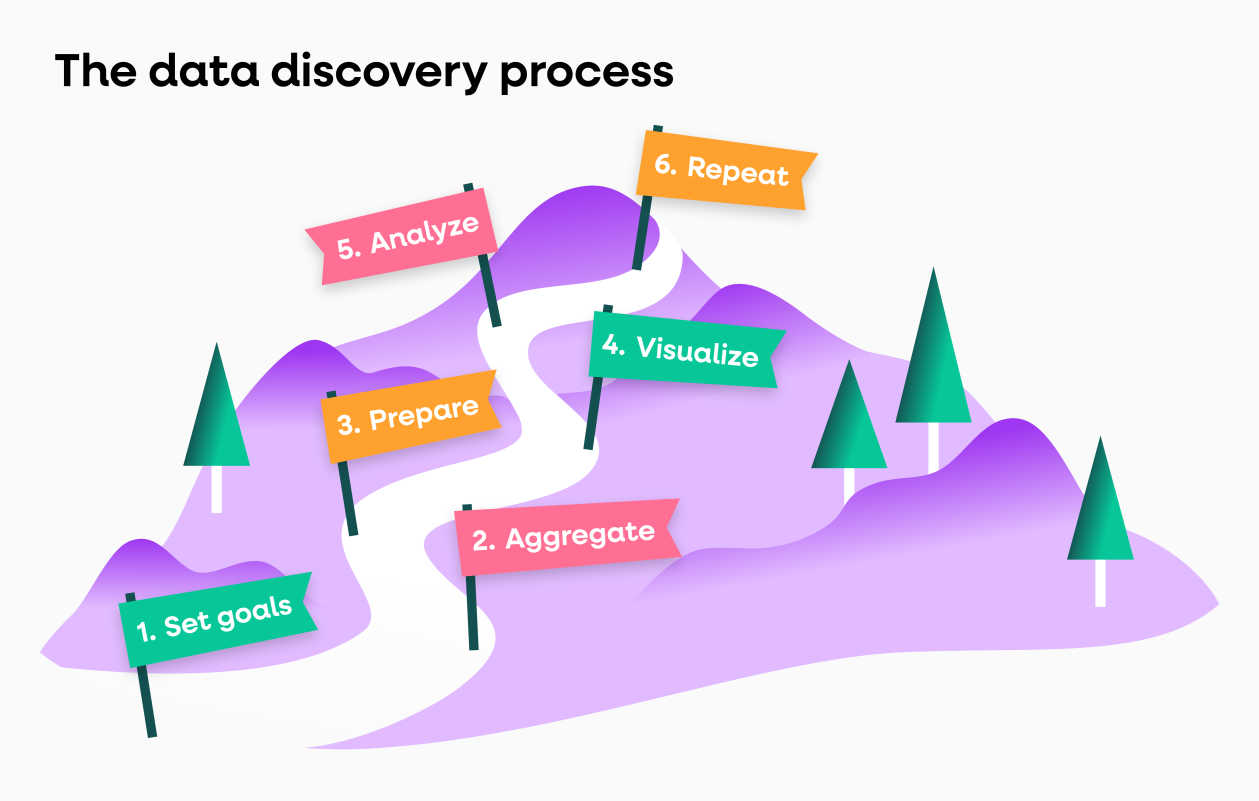
Although the process might vary slightly between organizations, it typically comprises six main steps:
1. Set goals
Clear goal setting ensures the data discovery process aligns with business objectives and focuses on uncovering relevant insights. Without well-defined goals, data discovery efforts risk losing relevance and delivering insignificant business value. Think of this step as setting the compass for your data treasure hunt!
2. Aggregate
The next step is to aggregate data, which involves collecting raw data from multiple sources and presenting it in a summarized format for higher-level analysis. The collection phase extracts data from sources and stores it as atomic data, the smallest unit of data.
3. Prepare
Data preparation is like prepping ingredients before cooking. This step involves cleaning the extracted data, applying formatting rules, and discarding irrelevant data. Standardizing data during this phase ensures uniform formatting, which aids in subsequent analysis and visualizations.
4. Visualize
Data visualization is the process of transforming complex data into charts, graphs, and maps, providing broader insights and convenient platforms for visual analysis. This step is essential in making insights easily understandable and actionable, fostering effective decision-making.
5. Analyze
Now comes the analysis phase. Here, data is summarized, organized, and applied with descriptive statistics to highlight patterns, trends, and insights.
Advanced analytics and reporting tools summarize data into comprehensible reports that aid businesses in making informed decisions.
6. Repeat
The final step is to repeat the process. Data discovery is cyclical and requires constant updating to provide relevant insights based on the latest data, making it an ongoing task rather than a one-off event.
As business objectives evolve over time, so should your data discovery process.
The role of AI in enhancing data discovery
Artificial Intelligence (AI) is the secret sauce that takes data discovery to the next level. AI plays a vital role in:
Automating data processing tasks
Allowing for intelligent exploration
Advanced analytics and modeling
The generation of automated insights.
Smart data discovery tools leverage AI to automate and enhance the process of analyzing and documenting data. They enable natural language interactions and provide a more intuitive data discovery experience. These automated tools streamline the discovery process by:
Cleansing data
Detecting and correcting outliers
Standardizing data quality
Facilitating the integration of disparate data sources
Data governance strategies in data discovery
Safeguarding data is essential. Data governance strategies ensure data security, compliance, and risk management by implementing policies and practices aligned with privacy laws and ethical data handling guidelines. These strategies play a critical role in maintaining compliance during data discovery, from classifying data to ensuring data quality.
AI and machine learning tools complement manual stewardship by automating:
Metadata management
Data classification
Governance
Enforcing data lineage tracking and access controls
Thus, integrating data governance practices into data discovery enhances the trust and transparency of data across your organization.
Use cases and examples of data discovery
Data discovery isn’t confined to a specific industry; its applications are as diverse as the businesses that use it. Here are some examples of industries that benefit from data discovery:
Optimizing retail strategies
Retailers apply data discovery to:
Understand customer behavior
Refine marketing strategies
Refine inventory strategies
Craft personalized marketing strategies
Make informed decisions about inventory management
Insights into customer preferences and buying patterns derived from data discovery can guide retailers in these areas.
Streamlining financial operations
In the financial sector, institutions implement data discovery for tasks like analyzing client behavior to predict mortgage defaults and strengthen risk management.
The detection of non-compliance through data discovery can significantly improve risk management strategies in financial services.
Advancing healthcare outcomes
In healthcare, data discovery plays a pivotal role in enhancing the accuracy of diagnoses, the effectiveness of treatments, and the overall improvement of patient care. Life sciences companies apply data discovery to analyze genomic data, clinical trial results, and drug interactions, thereby accelerating drug discovery, advancing personalized medicine, and contributing to healthcare research.
Achieving data literacy across the organization
Achieving data literacy across an organization is akin to equipping every employee with a superpower. Here are some strategies to enhance data literacy:
Organize educational workshops to provide personalized guidance and hands-on experience.
Implement online courses to allow employees to learn at their own pace.
Discuss a broad range of data-related topics to foster a deeper understanding. Implementing these strategies can enhance data literacy and empower employees with valuable skills.
AI data catalogs foster collaboration and facilitate access to data analysis for non-IT professionals, promoting a data-driven culture within organizations. Assessing literacy skills through tests and encouraging practice with real-world data sets helps individuals appreciate data’s complexities and nuances.
Discover your data with Fullstory
Now we understand data discovery is an iterative process involving collecting, analyzing, and visualizing data to identify patterns and insights. These insights are crucial for improving customer experiences, driving product innovation, optimizing operations, and guiding strategic decisions. Enhanced by AI infrastructure, effective data discovery is key to informed decision-making and robust business performance, positioning your organization for success.
But how do we leverage this process successfully? Fullstory’s behavioral data platform offers a sophisticated solution that further enhances your discovery capabilities. By emphasizing the detailed sentiment behind every user interaction on your website or application, Fullstory extends the reach and depth of data analysis.
Fullstory excels in capturing extensive user behavior data. This capability, in turn, enriches analytics and provides invaluable insights beyond traditional data examination methods.
Frequently asked questions
What is data discovery?
Data discovery involves collecting, analyzing, and visualizing data to uncover insights that inform decision-making and enhance business performance.
Why is data discovery important?
Data discovery is important because it enhances customer experiences, drives product innovation, optimizes operations, and informs strategic decisions. It's crucial for overall business success.
What are the methods of data discovery?
The two main methods of data discovery are manual and automated. Automated methods use AI and machine learning for more efficient analysis.
What is the role of AI in enhancing data discovery?
AI enhances data discovery by automating tasks, enabling intelligent exploration, and providing advanced analytics and insights. This can lead to more efficient and effective data analysis.
How can data discovery improve healthcare outcomes?
Data discovery can improve healthcare outcomes by enhancing diagnosis accuracy, treatment effectiveness, and overall patient care.