What if your analytics could tell you not just what users do but how they feel while doing it?
While traditional metrics show you the what, sentiment analysis utilizes data to reveal the why—turning customer interactions into insights that drive better business decisions.
Understanding sentiment analysis
Sentiment analysis is the process of using AI and natural language processing to determine whether text contains positive, negative, or neutral emotions. However, for businesses, this translates into a powerful way to understand how customers truly feel about your product or service by analyzing everything from support conversations to behavioral signals on their products—revealing the emotional story behind every interaction.
Picture your analytics dashboard. It tells you about completed purchases, abandoned carts, and time spent on the page. But beneath these metrics lies a deeper story—the emotional journey of your users. Sentiment analysis helps you understand that journey.
Why it matters now
Your customers share their feelings in two key ways: what they say and what they do. They're constantly leaving both explicit feedback and subtle behavioral clues across multiple channels:
Direct communication:
Customer support conversations
Social media interactions
Product reviews
Survey responses
Chat transcripts
Behavioral signals:
Frustration during checkout flows
Repeated clicks on navigation elements
Frequency of error messages
Patterns of feature usage
Session abandonment points
Every single interaction contains valuable emotional context that, when properly analyzed, can transform your understanding of the customer experience. By systematically capturing these insights, you can make informed decisions that directly impact customer satisfaction and business outcomes.
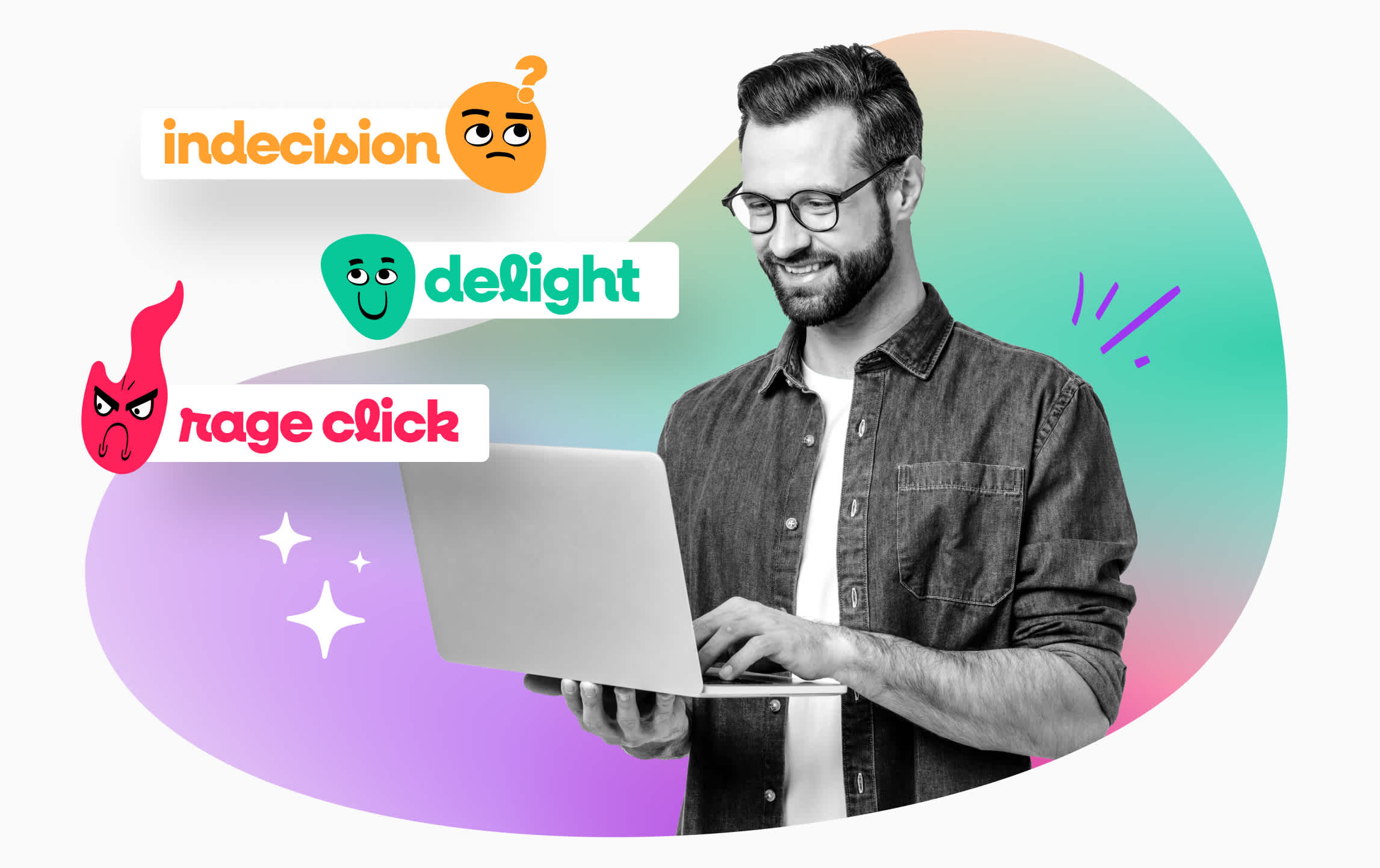
How customer sentiment analysis works
Understanding customer sentiment goes beyond just analyzing what people say—it's also about interpreting what they do. Modern sentiment analysis combines traditional text analysis with behavioral signals to paint a complete picture of customer experience:
Gathering customer signals
Your customers are constantly telling you how they feel, whether through direct feedback or their behavior on your site. By capturing both traditional and behavioral signals, you get the full story of their experience:
Traditional signals: Social media comments, reviews, support tickets, survey responses
Behavioral signals: Rage clicks, error clicks, form abandonment, scroll patterns
User interactions: Time spent on pages, navigation patterns, feature engagement
Processing and analysis
Making sense of this behavioral data requires sophisticated processing to understand explicit feedback and implicit behavioral cues. Here's how the system transforms raw data into meaningful insights:
Text data gets normalized and mapped to emotional indicators
Behavioral patterns reveal satisfaction or frustration
Machine learning identifies patterns in both text and behavior
Rule-based systems catch common frustration signals
Creating the complete picture
When traditional feedback and behavioral signals come together, you better understand your customer experience. This comprehensive view enables you to:
Detect frustration before it becomes a negative review
Identify pain points through both feedback and behavior
Understand the emotional journey behind interactions
Spot opportunities before they impact satisfaction
Combining what customers say with what they do, modern sentiment analysis reveals insights that might never appear in a survey response.
Sentiment analysis techniques
Before diving into advanced sentiment analysis, it's important to understand the fundamental approaches that power these capabilities. These techniques form the foundation for understanding and interpreting customer sentiment at scale.
Core analysis methods (how it's performed)
1. Rule-based analysis
The most straightforward and transparent approach to sentiment analysis relies on predetermined rules and dictionaries. Like a sophisticated spell-checker for emotions, it scans text for known positive and negative words to determine overall sentiment.
Uses predefined lexicons and rules
Counts positive and negative word occurrences
Best for: Small-scale, straightforward analysis
Limitations: Can miss context and sarcasm
2. Machine learning approaches
When sentiment gets complicated—think sarcasm, industry jargon, or subtle emotional cues—machine learning steps in. These sophisticated models learn from examples to understand context and nuance in ways rule-based systems can't.
Uses models like BERT, GPT, and LSTM
Learns patterns from labeled datasets
Handles complex, contextual analysis
Processes large volumes efficiently
3. Hybrid solutions
Why choose between rules and machine learning when you can have both? Hybrid approaches combine the transparency of rule-based systems with the sophistication of machine learning, offering the best of both worlds.
Combines rules and machine learning
Balances simplicity with sophistication
More accurate than single approaches
Requires more implementation effort
Advanced analysis types (what we're analyzing)
Building on these core techniques, advanced sentiment analysis provides deeper insights into customer feelings and behaviors. Each type serves a specific purpose in understanding the complete customer experience.
1. Polarity analysis
Think of polarity analysis as your customer sentiment thermometer. Instead of just measuring hot or cold (positive or negative), it captures the full spectrum of customer feelings, from very negative to very positive.
How it works:
Measures sentiment intensity across a spectrum
Creates scaled sentiment scores
Enables trend tracking over time
2. Emotion detection
Customer sentiment analysis typically identifies positive/negative feelings, but emotion detection goes deeper:
Delight (enthusiastic engagement, sharing)
Satisfaction (smooth completion, returns)
Trepidation (hesitation, multiple reviews)
Frustration (repeated actions, abandonment)
Anger (error clicks, rage clicks)
Rage (rapid repetitive actions, session abandonment)
How it works:
Combines language analysis with behavioral signals
Uses natural language processing for text analysis
Identifies multiple emotions per interaction
Maps emotional patterns across user journeys
3. Aspect-based analysis
Not all feedback is created equal. Aspect-based analysis helps you understand which specific features or aspects of your product drive certain sentiments. This granular understanding enables targeted improvements where they matter most.
Business value:
Feature-specific feedback analysis
Targeted improvement opportunities
Detailed product insights
4. Intent analysis
Understanding sentiment is good; predicting customer behavior is better. Intent analysis combines traditional sentiment analysis with behavioral signals to understand not just how customers feel, but what they're likely to do next.
Practical applications:
Purchase likelihood assessment
Support need prediction
Experience optimization
Choosing the right approach
Selecting the right combination of sentiment analysis techniques isn't just about technical capabilities—it's about matching your specific business needs with the right tools. When making your choice, consider your data volume, accuracy requirements, technical resources, and implementation timeline.
The most effective sentiment analysis strategies often combine multiple techniques and types, creating a comprehensive view of customer sentiment while managing technical complexity and resource requirements.
Tools for sentiment analysis: Make sense of what your customers feel
Every customer interaction tells a story. The key is having the right tools to understand what those stories mean for your business
Behavioral Data Platforms
Sentiment analysis tools like Fullstory reveal sentiment through user behavior—capturing the frustration in repeated clicks, the confusion in abandoned forms, or the satisfaction in smooth completions. By analyzing these digital body language signals, you can:
Spot friction before it becomes feedback
Identify patterns in user behavior
Understand the emotional journey behind every interaction
Take action on insights in real-time
Additional sentiment analysis tools
Different business needs might call for complementary approaches:
Social listening platforms help you monitor brand perception and customer sentiment across social media channels in real-time. They're particularly valuable for brands wanting to stay ahead of potential issues and engage with customers where conversations are happening.
Leading solutions include:
Brandwatch: Enterprise-level social listening with advanced sentiment analysis
Sprout Social: Combined social management and sentiment tracking
Customer feedback analytics focuses on analyzing direct customer feedback through surveys, reviews, and support interactions. They help transform qualitative feedback into quantitative insights, making it easier to analyze and act.
Popular tools include:
SentiSum: Specializes in support ticket analysis and customer feedback
Text iQ: Advanced survey analysis with customizable sentiment scoring
Integrated solutions combine multiple data sources into a single platform for businesses needing a comprehensive view of customer sentiment. These tools help connect various customer touchpoints for a more complete understanding.
Market leaders include:
Fullstory: Combines behavioral analysis with quantitative insights
Qualtrics XM: Combines experience and operational data
Medallia: Unified platform for customer experience management
The key is choosing tools that match your specific needs—whether that's understanding behavior, analyzing feedback, or both. Consider factors like:
Data volume and sources
Integration requirements
Team capabilities
Specific use cases
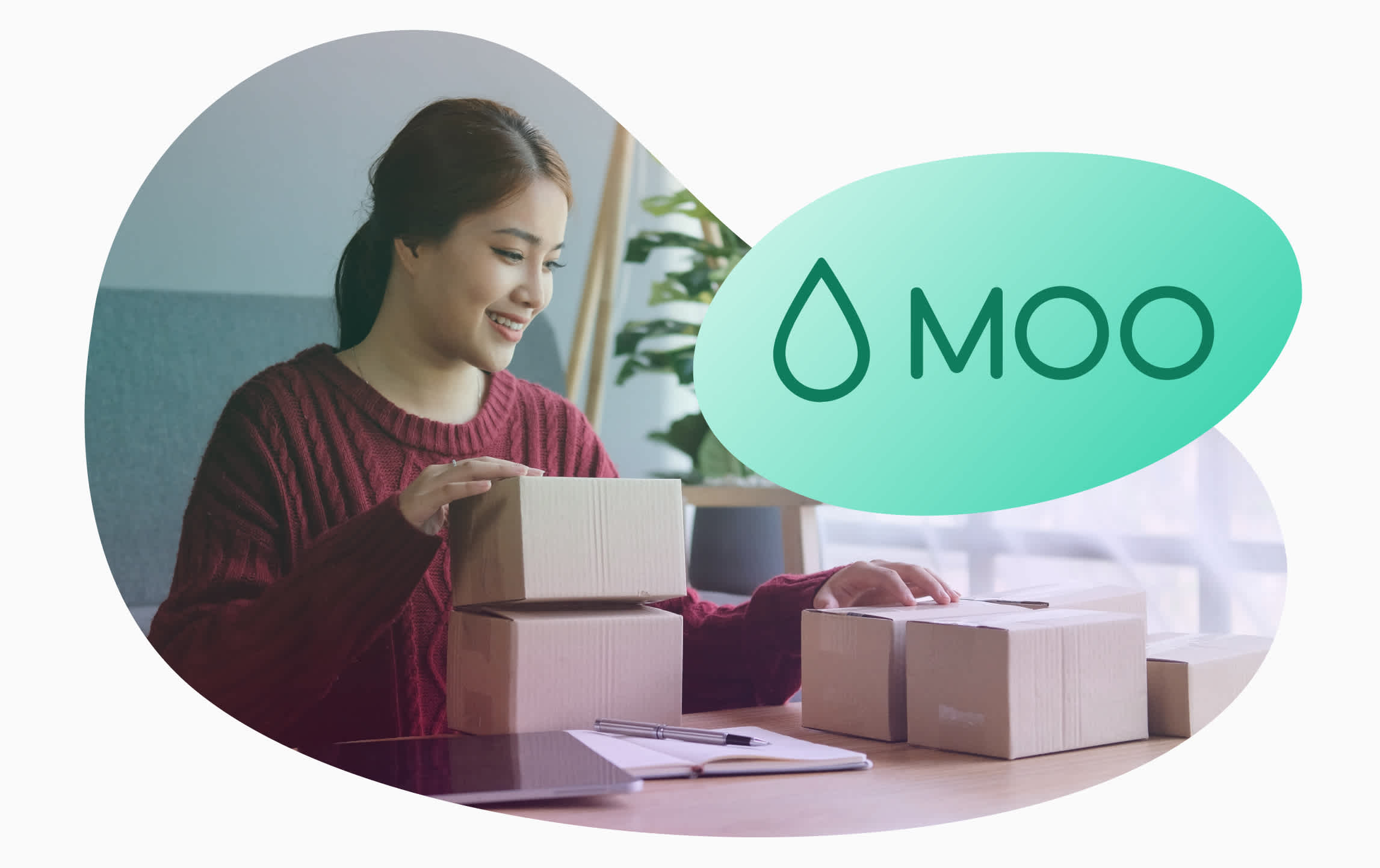
How MOO decoded customer sentiment to boost conversions
When MOO, a leading print and merchandise company, launched their self-serve branded merchandise platform in early 2024, they needed more than just analytics—they needed to understand the emotional journey of their customers.
By using Fullstory to analyze behavioral sentiment signals, they uncovered crucial insights about user frustration and satisfaction points.
The impact of understanding customer sentiment was significant:
67% reduction in user friction on beta product pages
12% decrease in checkout abandonment
12% increase in product engagement
Measuring customer sentiment
Customer sentiment goes beyond traditional experience metrics to reveal how customers truly feel about your product or service. While standard CX metrics like CSAT and NPS provide valuable insights, sentiment measurement adds another dimension to understanding your customers.
Understanding customer sentiment requires a multi-faceted approach. By combining traditional metrics with behavioral signals and sentiment scoring, you can build a more complete picture of your customers' emotional journey.
Here's how these different approaches work together:
Traditional metrics enhanced by sentiment
CSAT scores combined with sentiment context
NPS responses analyzed for emotional content
Customer Effort Score (CES) with sentiment indicators
Behavioral signals that reveal true feelings
Sentiment scoring
Think of sentiment scores as the emotional temperature of your customer interactions:
Positive: Indicating satisfaction and loyalty
Neutral: Showing standard engagement
Negative: Revealing potential issues or friction
Behavioral indicators
Actions often speak louder than survey responses:
User interaction patterns
Session behavior analysis
Feature engagement levels
Response to changes or updates
By combining these measurement approaches, you can get a more complete picture of not just what customers say but also how they truly feel about their experience with your product.
Turn customer sentiment into your competitive edge
Understanding how your customers feel isn't just nice to have—it's essential for business growth. Sentiment analysis transforms scattered feedback into actionable insights, helping you spot trends, solve problems, and create experiences that keep customers coming back.
The path to effective sentiment analysis is clear: start with the basics, use the right tools for your needs, and, most importantly, act on what you learn. Whether you're tracking social media comments, analyzing support tickets, or monitoring user behavior, every piece of feedback is an opportunity to strengthen your connection with customers.
Remember: Your customers are telling you how they feel in every interaction. Are you listening?
Ready to understand the true voice of your customers? See how Fullstory's behavioral data platform can help you decode customer sentiment and transform your digital experience. Get a demo today →